Intelligent Phenotyping (iPhen)
A phenotype refers to an individual’s observable traits whereas genotype refers to the genetic make up of an individual. In livestock, aquaculture, and plant industry sensors are being widely used to measure different phenotypes. For example, sensors are used to measure heart rates in oysters, to measure flow of water through plants, and behaviour patterns in cattle. These sensors generate a big volume of time series data and demands automated analysis for exhaustive and meaningful analysis of the underlying problem. Relevant industries are also interested in the relationship that exists between a particular phenotype of interest and the corresponding genotype (or another phenotype) for a set of individuals. The project aims to undertake research into the development of an analytical platform to assist in understanding different genotype-to-phenotype and phenotype-to-phenotype relationships.
The analytical platform developed in this project will assist in finding answers to the following key research questions in agriculture: (i) how accurately can we detect cattle behaviour and heat event from collar sensors, (ii) what are the appropriate phenotypes to infer the disease resistance of sheep?, (iii) what are the unique transcriptome regions (genotype) in infective amoebas that are responsible for gill disease (phenotype) in salmon? (iv) can we improve the accuracy of genotyping with pooled DNA samples through calibration methods? (v) is it effective to use quantitative genotypes (pooled DNA samples) to compute genetic breeding values? This project is a continuation of the work completed in the Aquaculture Molecular Informatics (AqMI) project with added focus on the cattle and sheep industry.
In order to address the above research questions, the following research areas will be explored in the ICT domain:
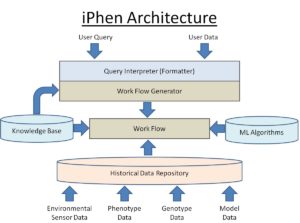
Iphen Architecture
Machine Learning for Time Series Data: Machine learning will be used to develop new approaches to detect the phenotype of animals with lower complexity and cost. The phenotype of parasite resistance/susceptibility in sheep has been previously collected by counting parasite eggs in faecal matter, a labour intensive and unpleasant task. A new approach to detect this phenotype from a single blood sample of a sheep is being considered. Machine learning based models will be developed to identify non-linear interactions between blood cell parameters and the phenotypes using blood samples collected during experiments. One of the unique features of this multi-variate time series analysis is that the number of blood samples is far smaller than the number of blood cell features. Consequently, in small, high dimension data-sets such as this, models will tend to be overfit and not provide a provide a sufficiently general solution. In addition, field based testing of sheep requires the phenotype to be identified using a single blood sample collected at any point in the infection cycle. This is challenging, given the variation in blood cell patterns across the infection life cycle and different animals that have been previously exposed or not exposed to the parasite. The time series data anlysis platform will also assist in analysis cattle behaviour.
Ensemble Learning: The measurement of pooled DNA samples are notoriously inaccurate, but it is far more cost effective to use pooled DNA samples in genomic association studies. Having pioneered ensemble based machine learning approaches to correct pooled DNA samples, the project will now examine the efficient calibration of far larger pooled data sets generated by the Illumina genotyping platform. Corrected data will allow our partners to better understand genetic associations to phenotypes and will inform future study design.
Machine Learning for Big Data: The genotyping chips used to compute the breeding capabilities of animals from pooled DNA samples are significantly large. This requires learning algorithms to be applied on big data. Dividing large data sets into multiple clusters, learning each data set separately and later combining them, while preserving the identical mapping capability of the whole data set is a research challenge in Big Data and this project will address that.
Ontology based Similarity Measures: In order to find similar transcriptome regions of an infective amoeba, gene ontology based descriptors will be utilised. Finding similarities between descriptors based on an ontology is an open research problem that the project will address.